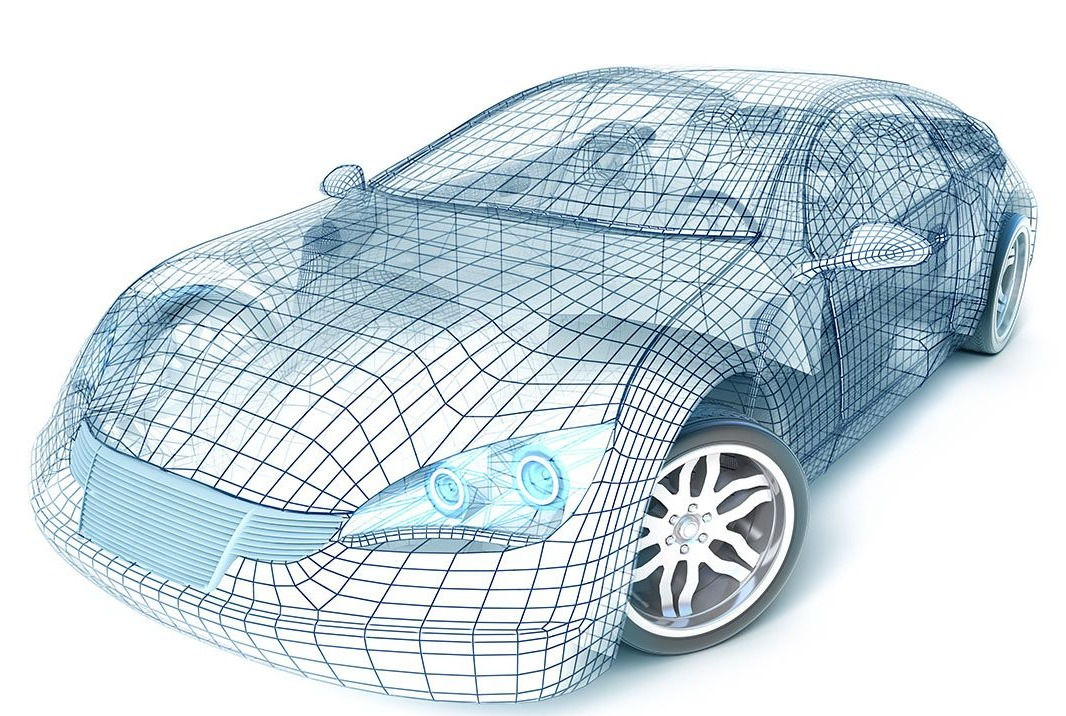
Deep material network uses a collection of connected simple building blocks with analytical homogenization solutions to describe complex overall material responses [Liu et. al CMAME 2019]. By discovering a proper topological representation of RVE with fewer degrees of freedom, this intelligent material model is believed to open new possibilities of high-fidelity efficient concurrent simulations for a large-scale heterogeneous structure. It also provides a mechanistic understanding of structure-property relations across material length scales and enables the development of parameterized microstructural database for material design and manufacturing.
​
​
Targeted applications: arbitrary RVE morphology, material nonlinearity (e.g. plasticity, damage), geometric nonlinearities (ex. large deformations).
With virtual material testing data from offline RVE analysis, the material network can be effectively trained using machine learning techniques, including
​
-
Stochastic gradient descent
-
Back-propagation algorithm
-
Similarity search
-
Automatic network compression
Illustration of deep material network
