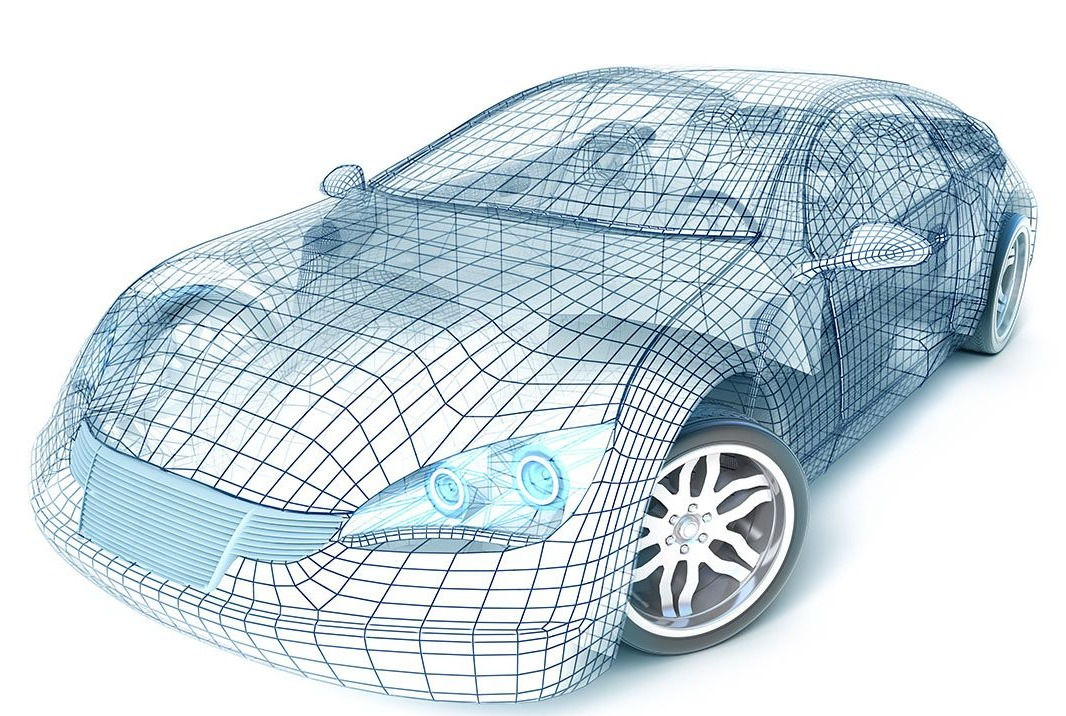
16 clusters
Self-consistent clustering analysis (SCA) is a novel reduced order model (ROM) technique [Liu et al. CMAME 2016]. It enables a powerful tradeoff between efficiency and accuracy through synergistic exploitation of high-fidelity analysis and efficient mean field homogenization using a data-clustering technique.
​
​
​
​
​
​
​
​
​
​
SCA starts with an offline stage, in which a database is created by high-fidelity RVE analysis. A data compression algorithm is then used to establish clustering groups with similar mechanistic features such as the local strain concentration factor. Material responses are assumed to be uniform in each cluster, and the interaction tensors between each pair of clusters are computed based on the Green’s function method. In the online stage, the discretized Lippmann-Schwinger integral equation is solved using a self-consistent scheme.


16 clusters
16 clusters
256 clusters
256 clusters
2D RVE with circular inclusions embedded in matrix
3D RVE with spherical inclusions embedded in matrix
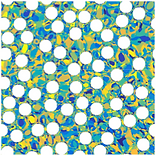